Most organizations misunderstand their own sales forecasting methodology — they don’t embrace their forecasts until it’s too late.
They assume sales forecasting is a leading indicator, because it’s literally predicting how revenue will change period over period — textbook leading indicator, right? Actually, forecasting is a lagging indicator because most people don’t decouple their forecast from plan and results.
You can’t wait until the period end is near to take impulsive actions when you realize you missed your target. That’s like getting on the scale only to do jumping jacks because you don’t like the number. It’s too late.
What is Sales Forecasting?
Sales forecasting is the use of expertise, historical data, sales activity data, and predictive analytics technologies to estimate the sales volume and subsequent revenue in a future time period. Nearly every sales team, and certainly every successful sales team, has a method for forecasting sales. A great sales forecast uses real-world data to estimate what outcomes are probable and does not rely on guesstimates. Sales forecasting is important because it can help guide resource planning and hiring, budget projections, risk management, and more.
Gainsight, for example, realized they were missing their quarterly forecasts and dug into the data to understand what went wrong. They discovered that 95% of deals that slipped did not have a meeting within two weeks of their forecasted close date. Gainsight’s reps had forecasted won deals but made no plans to actually close them.
It’s important then to understand that a sales forecast should be used to guide your sales planning process, not the other way around. But first, let’s discuss some of the methods commonly used to forecast sales.
Sales Forecasting Methods
We’ve structured our discussion of sales forecasting methodologies to start with the most basic and work our way up to the most complex. These also happen to correspond with the accuracy rates that can be expected from each forecasting method:
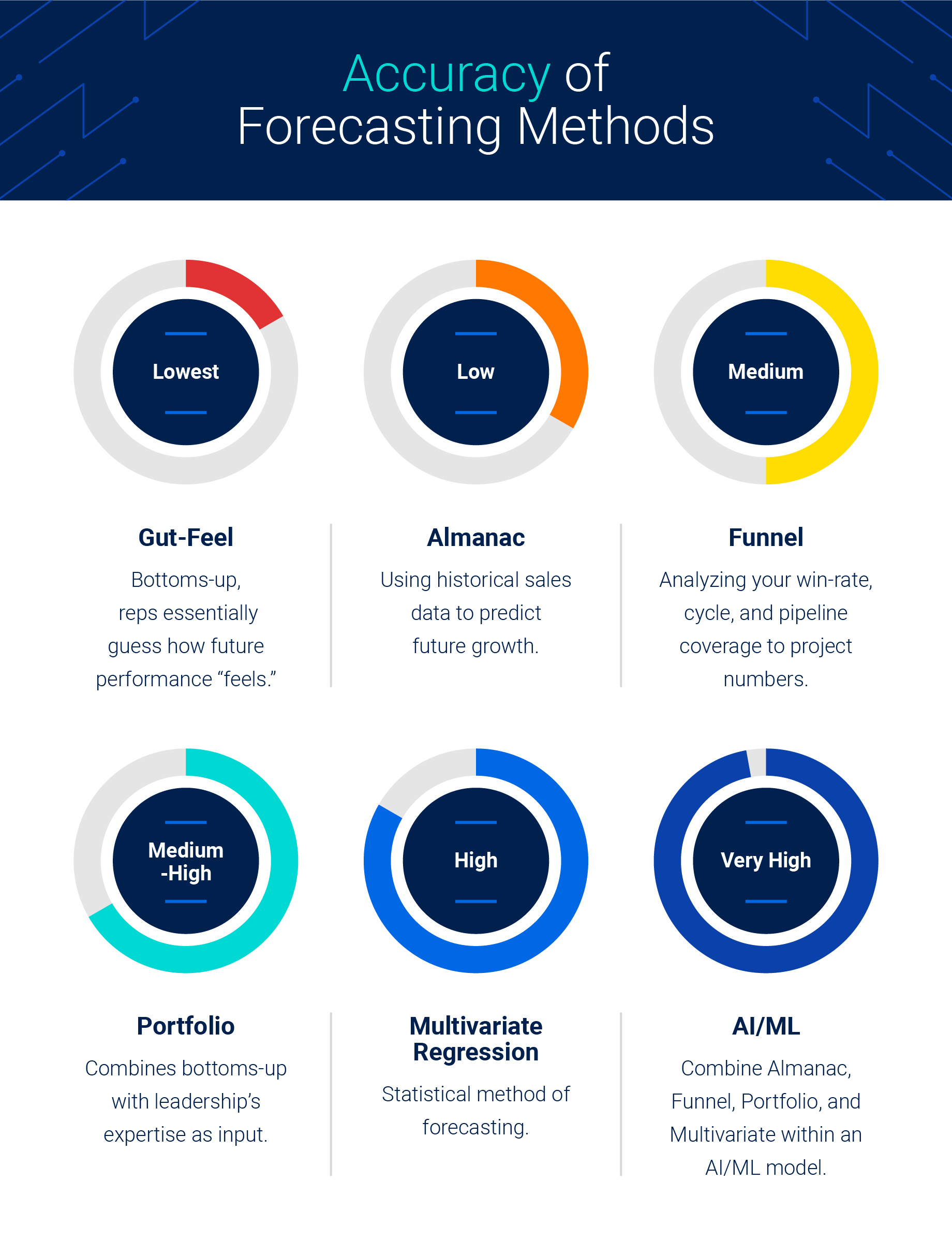
1. Gut-Feel Forecasting
A truly bottoms-up approach to forecasting is what we call the gut-feel forecast. Essentially, you ask your reps to gauge how they feel about their deals, how likely they are to close, and when they expect them to close.
This is the least accurate forecasting method and like flying an airplane with no instruments in 2021 — there’s no reason to take that risk. Deal slippage and swing deals can make these forecasts wildly inaccurate.
For example, as Q1 is coming to a close, the CFO has asked the sales manager to forecast sales next quarter. Based on anecdotal experience with Q2 being a stagnant sales period and recent memory of pipeline coverage, the manager presents a number that reflects his gut-feeling about performance next quarter.
The gut-feel forecast is a method that would typically be used by smaller sales organizations or teams with fewer deals, longer sales cycles, larger ACV and in more traditional industries. These companies rely on the experience of their sales reps and managers to predict the future. We don’t recommend this method but wanted to include a brief mention of it for completeness.
2. Almanac Forecasting Method
Using historical data to forecast future performance is what we call the Almanac method. The use of historical data and a projection of growth can be slightly more accurate than simply going with your gut feeling, but there are some downsides.
The almanac forecasting method doesn’t account for “climate change.” If there’s a shift in industry dynamics or macroeconomic volatility, the historical data might no longer be relevant. The year 2020 illustrates this problem, since forecasts built on 2019 data were rendered useless.
In this example, when the CFO approaches the sales manager to forecast Q2, the manager immediately looks back at performance data of the previous year’s Q2 performance compared to other quarters, as well as Q2 trends over the last decade. The manager also looks at the historical sales activity data for their reps to uncover trends in each member’s ability and cadence to close deals.
This method of forecasting is helpful for established companies with lots of historical data to pull from. The bigger the backlog of performance data, the more accurate the forecast is likely to be.
3. Funnel Forecasting
Understanding the performance of your funnel, such as your win-rates and average sales cycle duration, can help guide your forecasting methodology. We call this funnel forecasting.
For example, if your sales cycle is two months and your win-rate is 40%, on average, you can use this information to forecast based on your pipeline coverage. If you have ten opportunities worth $1 million each, a safe forecast for the quarter would be $4 million.
Funnel forecasting is heavily dependent on your pipeline’s coverage, health, and hygiene. The longer your sales cycle, the more vital this becomes because, as they say, pipeline cures all.
This method of forecasting is most useful when your deals are homogeneous in nature and therefore consistent and predictable based on their status in the pipeline.
4. Portfolio Forecasting
Similar to how a portfolio manager would use the financial data available to them to forecast performance and hedge against risk, a sales leader uses their experience and expert opinion paired with the sales data available to them to forecast.
This method pulls from the various forecasting strategies already discussed, combining to make for a more accurate forecast.
For example, a sales manager has assessed the historic sales data, reviewed the pipeline, discussed with her reps the likelihood of closing, and now she can combine her expertise with real data to forecast.
This method of forecasting can also help mitigate risk. For example, the manager, projecting a down quarter, could shift internal resources to focus heavily on upselling existing customers, knowing that this additional revenue would be more profitable and sustainable.
5. Multivariate Regression Analysis
Multivariate regression is a statistical method of sales forecasting that takes as input multiple variables that influence the desired output, typically revenue. The change in each variable will have a corresponding effect on the predicted output.
The multivariate regression approach relies heavily on data completeness and accuracy, since it uses these values to analyze previous and predict future performance. Multivariate regression is the foundation of machine learning — a topic we will dive into shortly.
In this example, the CFO comes to the sales manager who then consults her insights analyst to run a regression analysis. The analyst takes previous sales activity data and the results of the closed opportunity (won or lost) and can then analyze current sales activity data to plot how likely a given deal is to close-won.
Companies that use multivariate regression are typically more sophisticated and data-driven in their sales approach.
6. Machine Learning and AI
This brings us to the most sophisticated — and powerful — method for sales forecasting. Modern machine learning algorithms are trained on historical data and assigned weights and biases by the algorithm to learn the expected outcome. ML and AI learn from the data in ways even the developers are unaware of or don’t understand why.
Once the ML algorithm has been sufficiently trained and tested, data can be analyzed in real-time to make rolling forecasts and highly accurate predictions. Many ML models boast accuracy rates in the 99th percentile, with an understandable and acceptable margin of error.
As an example, you train a model on your sales team’s data including a few features (datapoints) like date, number of days from opportunity to close, contract value, and outcome. Training on this data, the model extracts information about seasonality, the impact of contract value on the sales cycle, and more which can be used to feed in similar data (minus the outcome) and forecast accurately.
The Value of Good Data for Great Forecasts
The biggest predictor of a sales forecast’s efficacy is the data used as the basis for the prediction. Even intuition-based forecasting (like gut-feel) methods require accurate information if you are to make accurate predictions.
Consider the difference between science and philosophy. Science uses objective data to prove theories and predict phenomena in the physical world while philosophy uses subjective reasoning. Which prediction would you rely on?
Data quality is usually measured on a few aspects including completeness, consistency, accuracy, and timeliness. When considering a traditional CRM, we put our faith in humans to manually update the database, which could result in the following hypothetical deviations from data quality:
- Completeness: Some fields are left blank because the rep was in a hurry and forgot to finish updating.
- Consistency: Some sales reps update once per day, others once per week, and others more sporadically, leading to inconsistencies when forecasting.
- Accuracy: In an attempt to sandbag expectations, a sales rep marked deals as qualification when they were actually in negotiations and likely to close.
- Timeliness: The rep has opportunities that have been in the pipeline for months and their contacts aren’t even with the company anymore.
In the past, reps would update their CRM at irregular intervals and with incomplete or inconsistent information. Using this data to forecast produces wildly inaccurate predictions and wide variations in numbers from period to period.
How to Reduce Forecast Variance
One way to reduce forecasting variance is to promote forecasting linearity within your team. Essentially, you break your plan down into smaller, more attainable goals which can be used to measure performance to plan.
Let’s say you have a rep forecasting next quarter to close $100k in month one, $100k in month two, and $800k in month three. This rep has a large amount of linearity risk in their forecast.
Our team uses the 20-30-50 rule to reduce linearity risk because $200k in month one, $300k in month two, and $500k in month three is likely to have a higher forecast accuracy and is less vulnerable to deal slippage in month three ruining the entire quarter’s forecast.
If you haven’t attained 30% of quota by the end of period two, you will have to adjust either your plan or your forecast.

How Machine Learning Methods Have Changed Sales Forecasting
When you have swing deals and large, lumpy deals, you can’t trust any of the forecasting methods we’ve discussed up to this point, but this is when ML and AI are game changers.
Machine learning in sales forecasting is the process of training a model to take sales activity data, learn how each input contributes to a weighted output, and use the model to predict outcomes based on previously unseen and real-time performance data.
You might now be comparing this to our multivariate linear regression discussion and looking for a difference. The difference is that ML algorithms usually have hidden layers, tweaking the weights and biases between neurons resulting in highly accurate predictions, for reasons often unknown even to the model’s creators.
ML models are being used to make sales forecasts more accurate and have changed the forecasting game in some of the following ways:
Focus on Performance, Not Reporting
Sales is the only profession where we ask people to report on their progress while they are mid-execution. Self-reporting via your CRM is also exposed to subjective differences between the data reports of one rep to another.
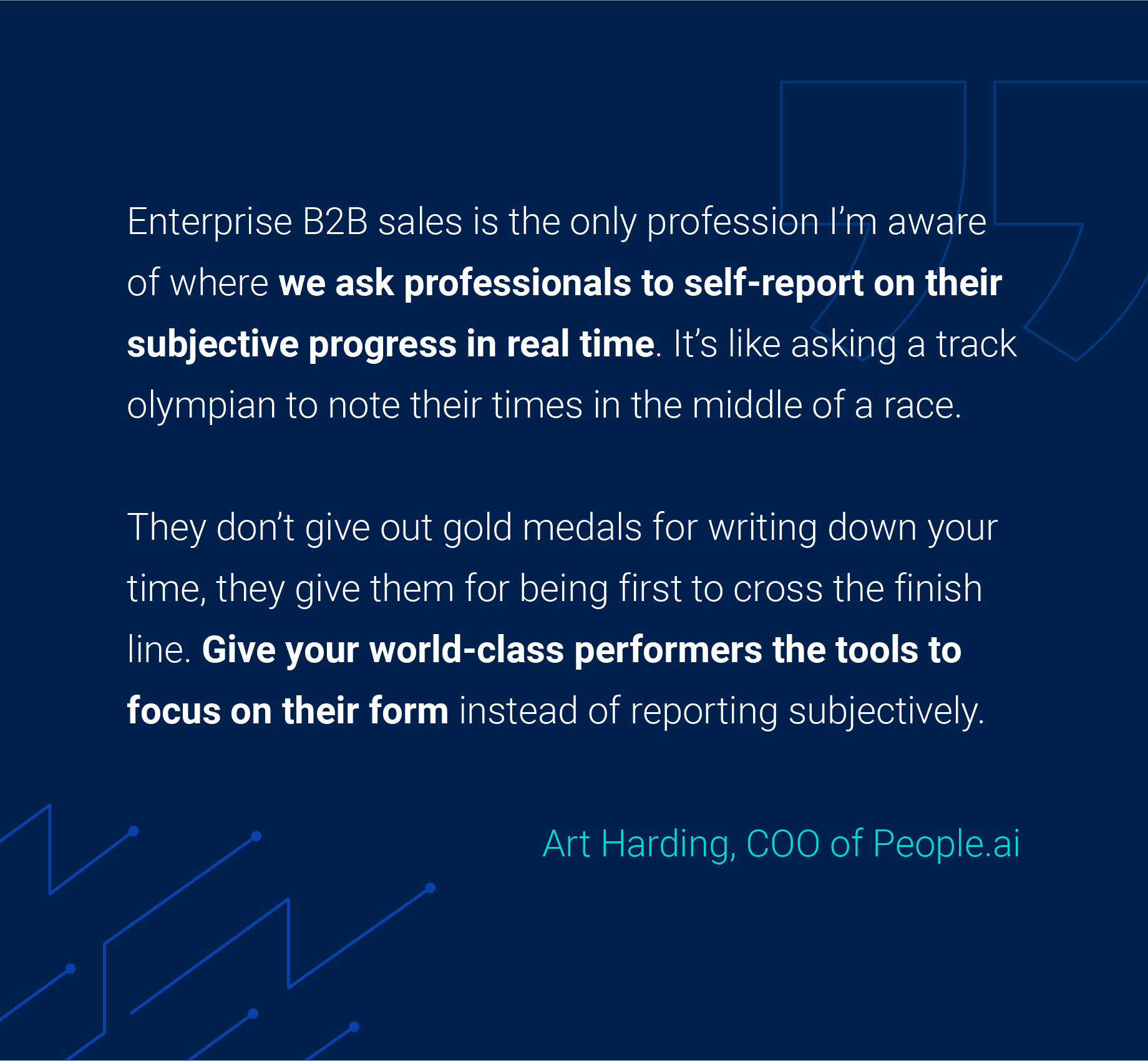
ML models, like those trained, tested, and optimized by People.ai, have enabled sales performers to focus on what actually moves the needle, rather than on reporting.
Uncover New Correlations and Recommendations
What machine learning offers forecasting, as opposed to forecasting with linear regression, is the ability for the models to uncover new trends in the data that may have previously gone unnoticed.
For example, let’s assume your regression forecast revealed a clear correlation between the length of time spent on the phone and an increase in win-rate. Providing more, seemingly unimportant data, to the ML model revealed that even more important than time spent on the call was making calls at regular intervals, even if the prospect didn’t answer.
Our hypothetical example would help your team become more structured in their cadence of sales calls, without feeling the need to drag conversations out for the sake of increasing the metric of time spent on the phone.
Increased Accuracy and Predictable Revenue
Machine learning models are not 100% accurate, but with a good ML model ranging between 95-99%+ accuracy on test data, you can have a great deal of confidence in the forecasts they produce.
When you train an ML model, you need a large quantity of quality data that is accurately labeled with the corresponding output. Before training, you take a subset of the data, often 10-20% to test the accuracy of the model on data that is already labeled.
Once the model has been trained and tested, the model can be applied to data with unknown outcomes and therefore generate a predictable revenue forecast.
Sales Forecasting with ML and AI
A methodological mindshift must be made to trust and rely on your AI-generated forecasts to coach your reps towards improved performance.
With automated, real-time insights into sales activity data using People.ai, you can reduce forecast variance among your reps, monitor their progress, and push them to improve with the recommendations surfaced from your data.
If you are looking to level up your sales forecasting methodology, consider working with your developers or data insights team to begin testing various machine learning models and find one that can make accurate predictions based on your team’s sales data.
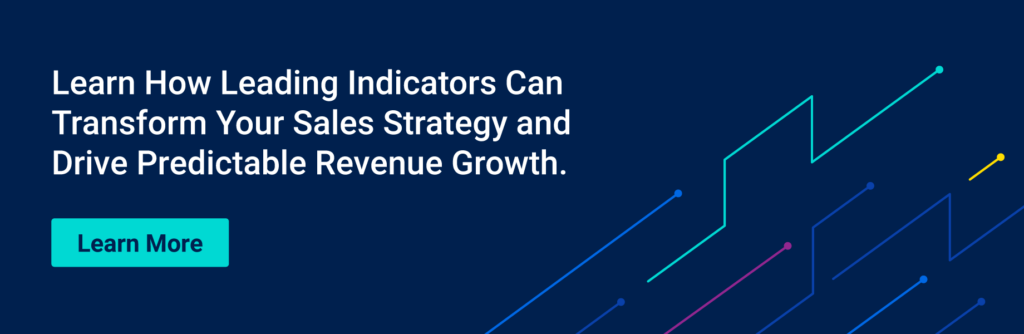