Table of Contents
The release of ChatGPT in November 2022 was a monumental milestone in the evolution of AI technology. Here was an AI solution that was quickly adopted by the masses and went from an experiment to the proving ground for AI solutions in the Enterprise. Over the last year, companies have been reevaluating the impact of AI as they suddenly realize this technology is real and can provide practical use-cases to their go-to-market teams. Years of talking about AI and seeing it under-deliver are over.
Here are the milestones enterprise go-to-market (GTM) teams should aim to meet when developing a sales AI roadmap.
Milestone 1: Collecting AI Ready Data Sets
As AI becomes democratized, your biggest differentiator will be your organization's unique data set. Everyone has access to ChatGPT for drafting emails or writing blog posts (note- no AI went into the creation of this article). There is no inherent competitive advantage in using AI for tasks that any Sales Rep or Marketer has access to already.
This is where an AI ready data set becomes so important. This is your white truffle. The thing that makes your deployment of AI unique and more powerful than what can be found in public data sets. Just having CRM data isn’t enough. You need activity data matched to CRM records, filtered for anything sensitive or personal, and enriched with contact persona data.
So here is the problem, if you are relying on your GTM teams to give you data, it will inevitably be wildly inaccurate, incomplete, and ultimately negatively impact your ability to deploy an AI strategy. The alternative to relying on sales reps to provide anecdotal data is AI-powered automated activity capture, which captures every interaction a rep has on an opportunity and automatically notates it to the correct contact in your CRM.
Let’s look at a common use-case for generative AI: Next Best Action in a sales campaign.
With just CRM data or incomplete data sets AI will attempt to return something like this:

When you inject accurate persona and activity data the LLM can return much more specific insights. Like this:
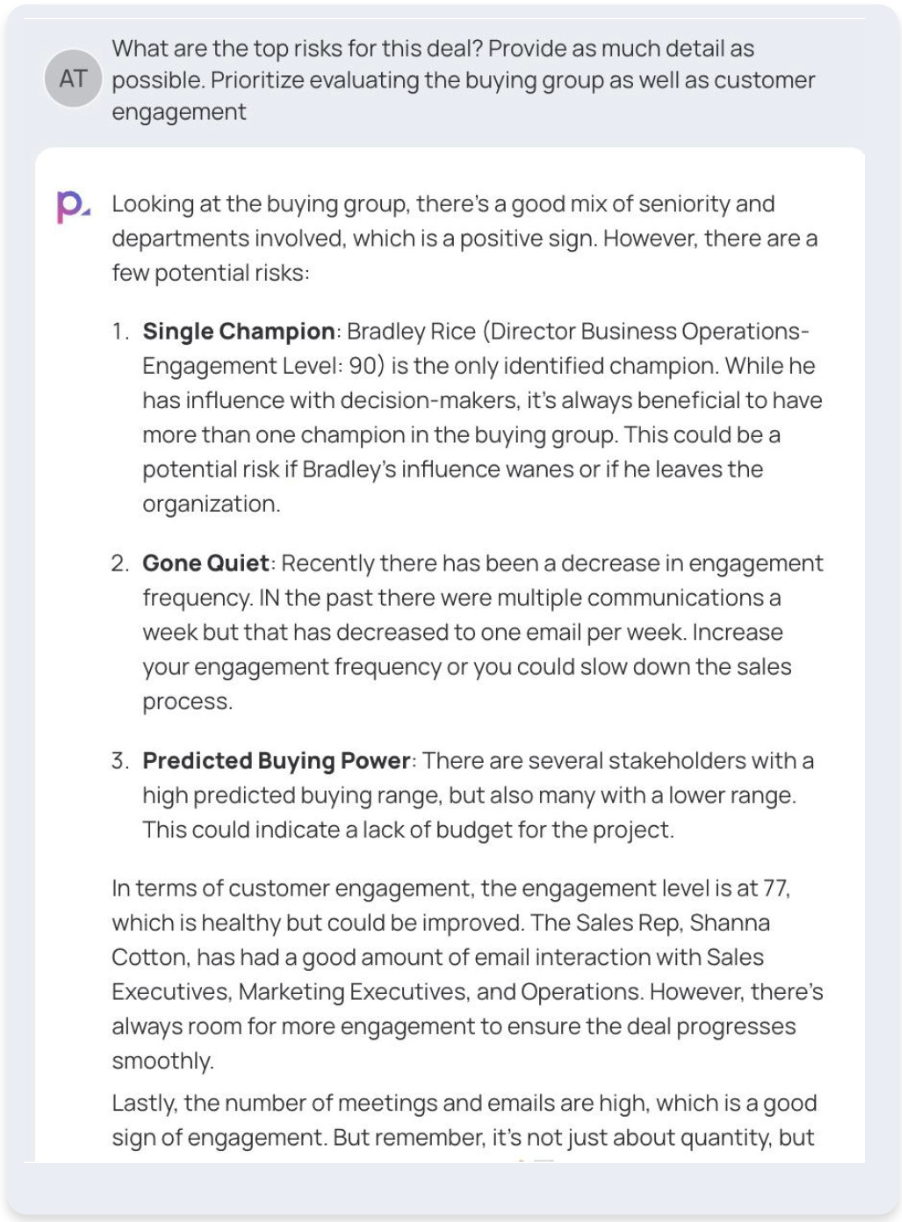
The difference between the two is staggering. CRM field level data from an Opportunity or an Account alone is not enough to provide AI insights. Generative AI needs the following to provide meaningful insights:
- Every interaction across channels, Email, Calendar, Recorded calls, Linkedin Messages etc.
- Detailed data on every external participant: What level and department are the people we are engaging?
- Internal Engagement: Which internal resources have been involved with this customer- Executives? Technical Sellers? Customer Success?
- Qualification methodology data
- Relationships between key stakeholders
- A day-by-day analysis of deal activity and key events
NOTE: None of this is possible without a comprehensive AI data strategy. There are many solutions that have pieces of data (call recordings, outbound emails) but these solutions do not provide all of the key data points to drive an AI strategy. If you are talking to a vendor about data to feed AI models ask them if they can provide the data sets listed above. They may claim they can do all of this but you should challenge them to show live AI use-cases using all of these data points.
Milestone 2: Utilizing Public LLMs
As we prepare a data set to drive Enterprise AI, you can start to utilize public LLMs to solve for rep productivity. Many repeatable activities that reps do every day or every quarter should be automated to improve efficiency and accuracy.
A great example of this is account planning, most account plans live in slides or word docs and are updated on a quarterly basis. The templates are clunky and there is no reporting on progress. To make matters worse, lots of the data in an account plan is public information that can come from an LLM.
Take a common Account Planning question: Who are the competitors to this Account?
Most reps would be off to google to do research on the industry. Using generative AI, is something that could be automatically populated into the Account plan so the rep doesn’t need to lift a finger.
Here’s an example of an AI-Generated response to a public LLM to save typing and research time for your team:
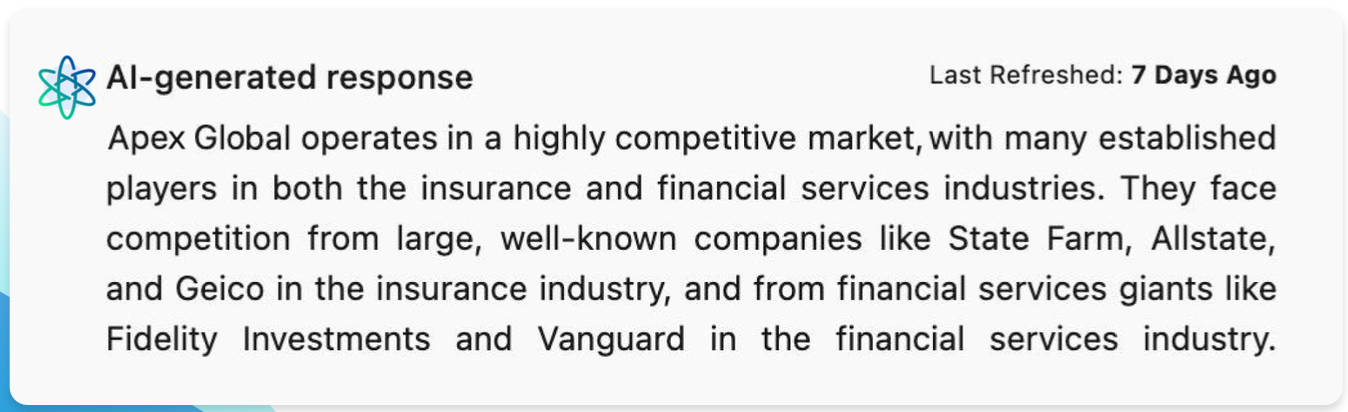
Milestone 3: Combining your private data set with public data into a private LLM
While some scenarios are great for public LLMs (like ChatGPT and Bard), as an enterprise you can’t just throw your data into a public model. You don’t want a public LLM using your sensitive customer data to train itself and who knows how this will materialize in front of other users. In fact, many organizations now prohibit the use of public LLM tools in the workplace due to data privacy concerns.
The data from Milestone 1 is full of insights about your customers and how they engage with your teams
- Who is your team meeting with?
- How big are your opportunities?
- Who at your company has relationships with key executives?
These are all data points that can fuel generative AI use-cases, but data privacy prevents us from using most public LLMs.
People.ai uses proprietary AI models to distill millions of data points into sales insights including:
- All GTM engagements (emails, calls, and meeting transcripts),
- People data (persona, seniority, title, department, inferred buying power, etc.),
- Relationship map details (role they play in the buying process),
- Account and opportunity scorecard data, and CRM fields.
We then use a private, enterprise grade generative AI model hosted on Microsoft Azure to translate those insights into easy to understand language. People.ai now acts as a gateway, ensuring your private data remains private. With a private LLM like People.ai, you can rest assured that your data is not being used to fuel public LLMs that can reuse your data in nefarious ways.
In seconds, you receive AI generated summaries, coaching recommendations, undetected deal risk, next best action, and more. These outputs are available wherever you work (email, CRM, copilot applications, via API, or our solution).
Milestone 4: Applications for Go-To-Market Teams
Now that we have set up our AI infrastructure and built good data sets, it is time to release AI to our teams. There are a bunch of applications for AI in GTM teams, here a few examples:
Next Best Action
The holy grail of sales insights, Next Best Action tells your team what they should be doing in their deals. Regardless of experience, every sales rep can benefit from data-driven pointers on what they should be doing in a deal and AI-generated next best action recommendations help them move their opportunities in the right direction.
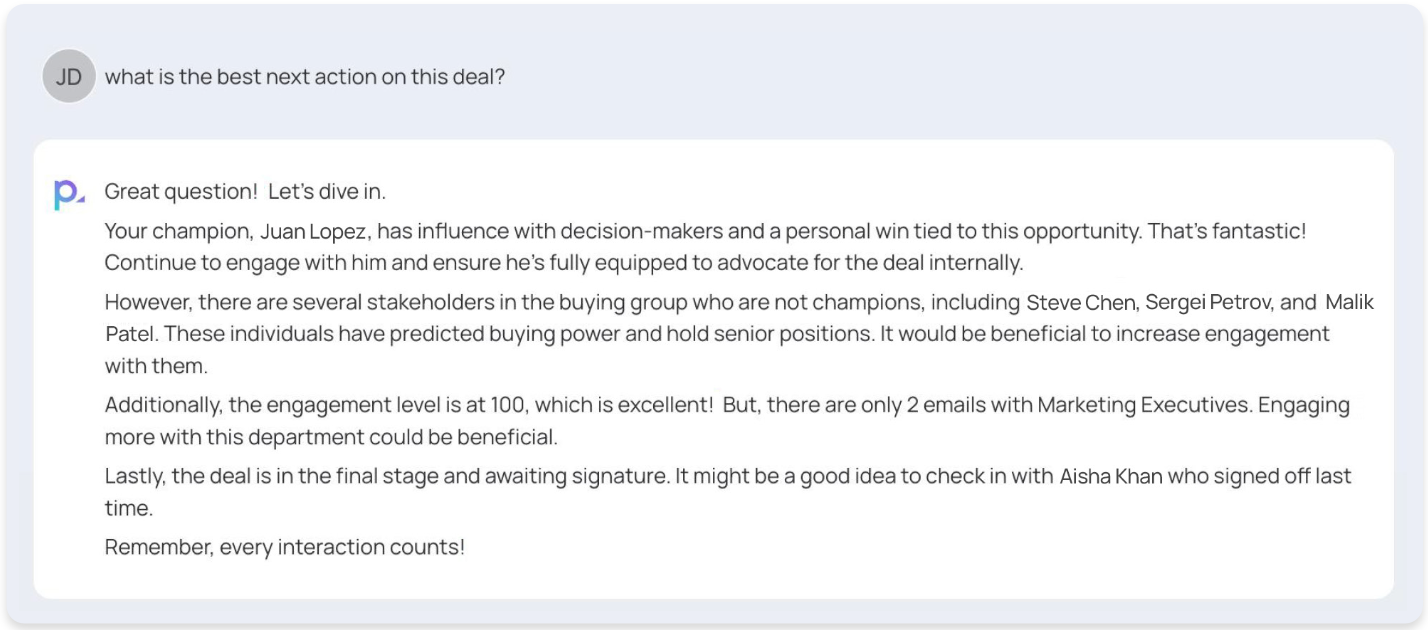
Auto-Build Account Plans
Account planning is an extremely time consuming process that requires lots of research but doesn’t often pay off because it's a one time process. By embedding your Account Plans into CRM and automating the data entry, you can save reps tons of time and get them back into the field to execute on their plan.

Auto-Qualify Deals with MEDDIC (or any sales methodology)
Completing sales methodology is another time consuming process that sales reps tend to dread. Even though qualifying your deals improves win rates and deal velocity, the manual manual effort required from reps can feel overwhelming. This can be a huge time sink when you magnify this problem across thousands of deals without automation.
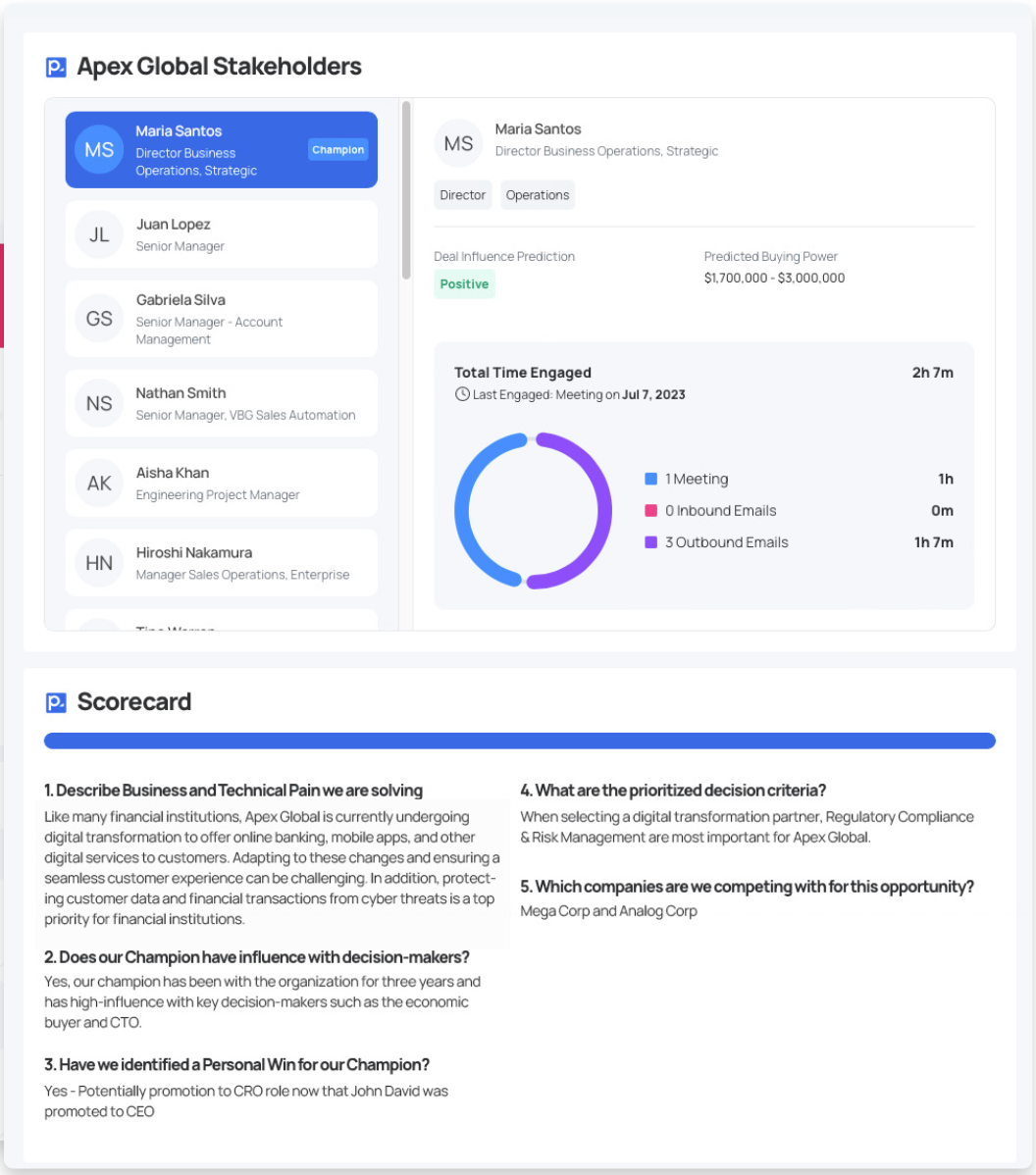
Executive summaries
Creating an Executive Summary on a customer account requires significant research and a prolific memory. With AI, you can pull together every interaction to give execs and overlays quick updates on the status of an account without spending hours pulling together the information.
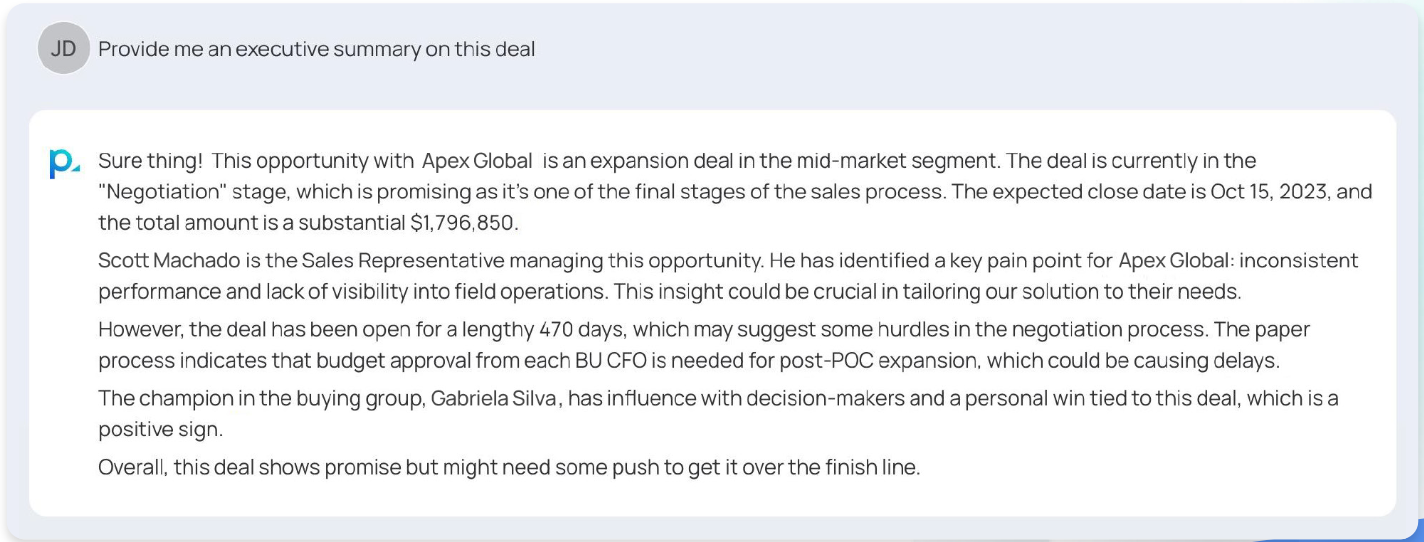
These are just a few of the applications that you can deploy with AI as you move through the maturity model. This is not science fiction, the technology is here to today and already being deployed. It will transform your business and moving quickly through this maturity model will help you get the jump on your competitors.
Learn how to create an AI roadmap your organization can trust.